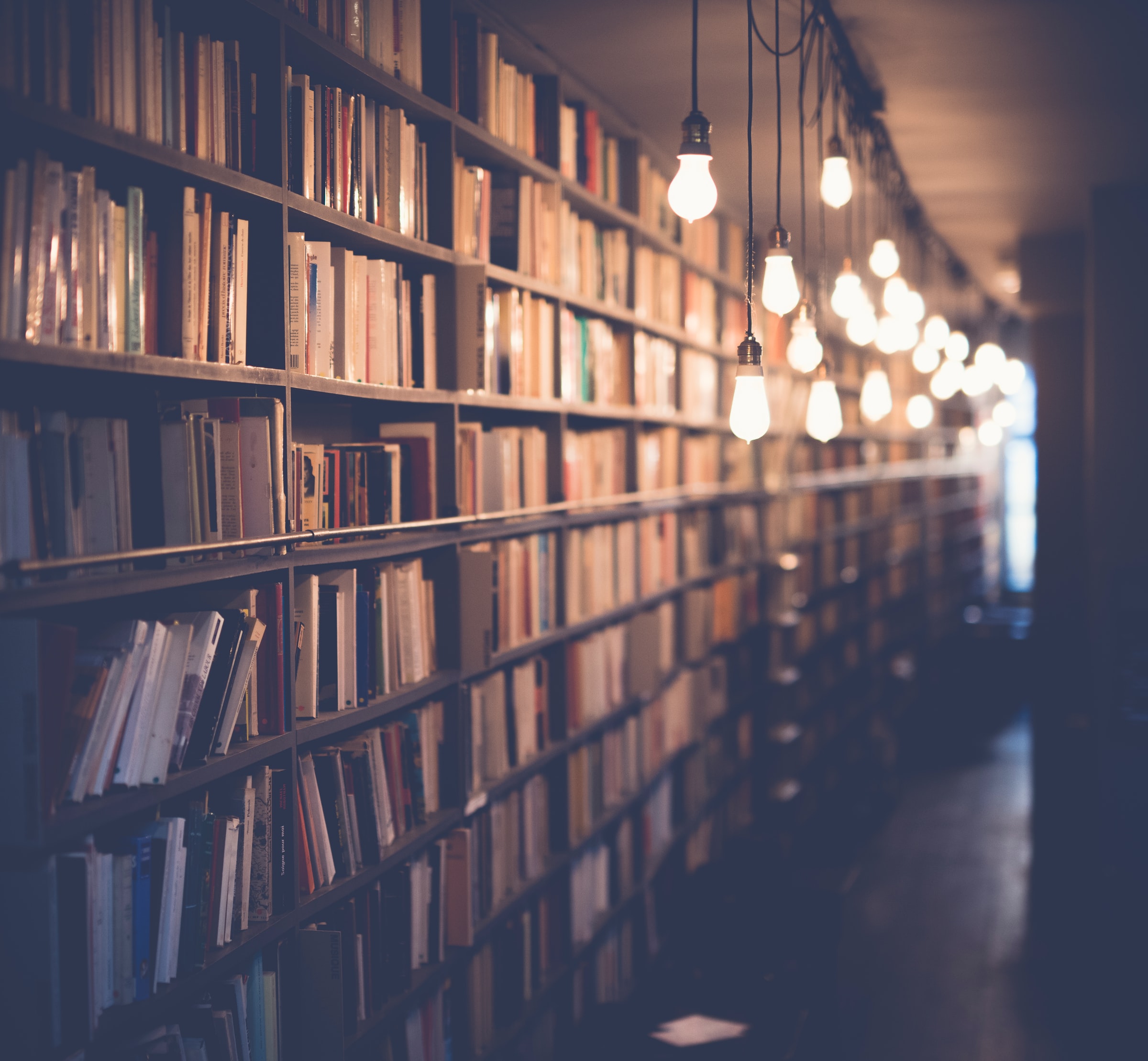
Associative classifiers are a family of classification techniques that use association rules to classify a new instance. Many works were proposed in this context, with a focus on how to select and combine the relevant association rules for classification. However, most of them do not consider the imperfection of data. In this paper, we tackle the problem of multi-rules based classification for evidential data, i.e., data where imperfection is represented and managed through the Dempster-Shafer theory. In this context, a new algorithm called WEviRC is introduced. This method uses different pruning techniques to eliminate the irrelevant rules and then to combine the selected ones using the powerful combination rules of the Dempster-Shafer theory. To evaluate our introduced method, we conducted extensive experiments on several data sets. Experiments show positive results in terms of classification quality and compare well with the existing methods.